The Defense Department has long used artificial intelligence to detect objects in battlespaces, but the capability has been mainly limited to identification. New advancements in AI and data analysis can offer leaders new levels of mission awareness with insights into intent, path predictions, abnormalities, and other revealing characterizations.
The DoD has an extensive wealth of data. In today’s sensor-filled theaters, commanders can access text, images, video, radio signals, and sensor data from all sorts of assets. However, each data type is often analyzed separately, leaving human analysts to draw — and potentially miss — connections.
RELATED
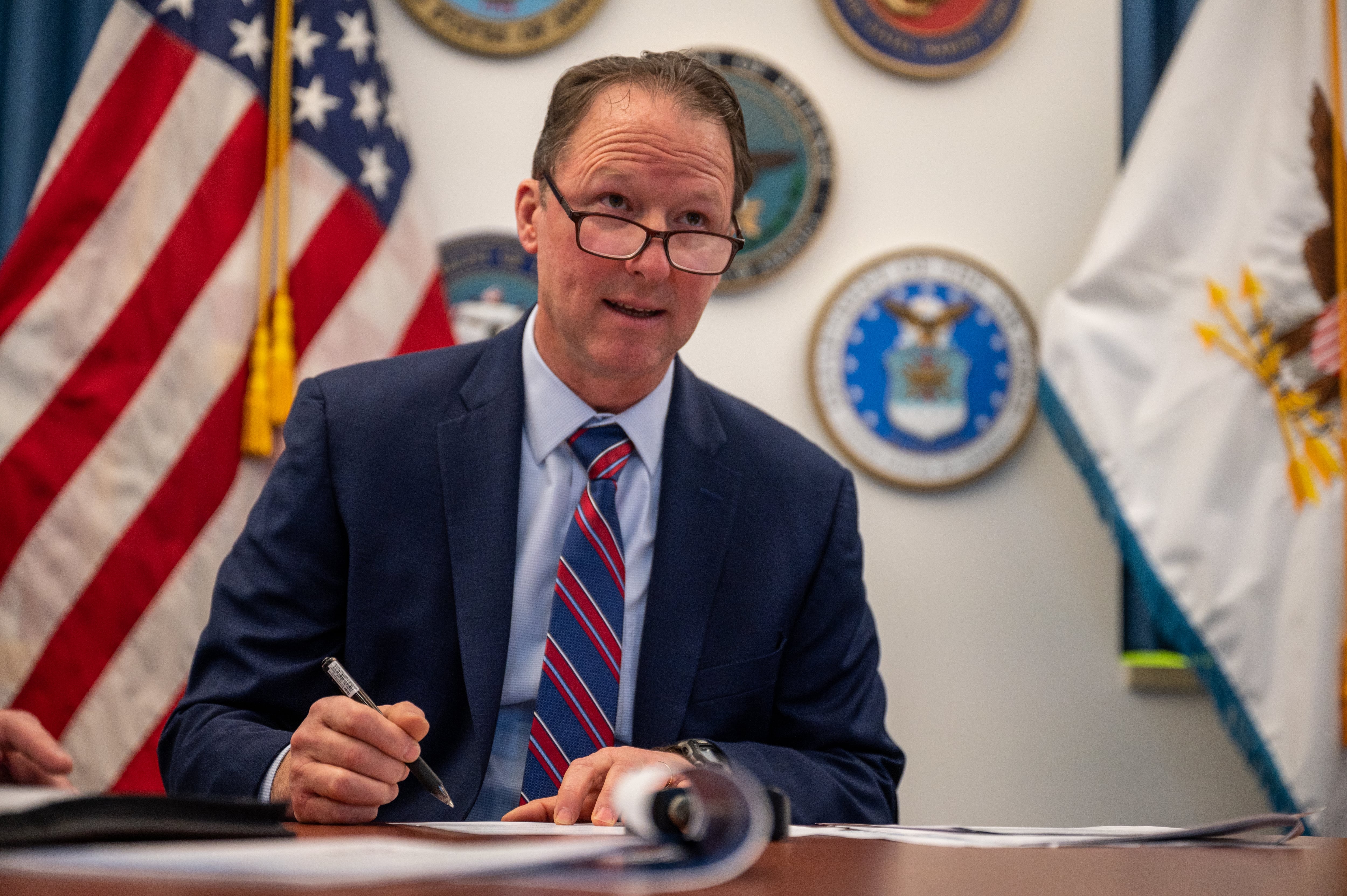
Using AI frameworks for multimodal data analysis allows different data streams to be analyzed together, offering decision-makers a comprehensive view of an event. For example, Navy systems can identify a ship nearby, but generative AI could zero in on the country of origin, ship class, and whether the system has encountered that specific vessel before.
With an object of interest identified, data fusion techniques and machine learning algorithms could review all the data available for other complementary information. Radio signals could show that the ship stopped emitting signals and no crew members are using cell phones. Has the vessel gone dark to prepare for battle, or could it be in distress? Pulling in recent weather reports could help decide the next move.
This enhanced situational awareness is only possible if real-time analysis happens at the edge instead of sending data to a central location for processing.
Keeping AI local is critical for battlefield awareness, cybersecurity, and healthcare monitoring applications requiring timely responses. To prepare, DoD must adopt solutions with significant computing power at the edge, find ways to reduce the size of their AI/ML models and mitigate new security threats.
With most new AI tools and models being open, meaning that the information placed into these technologies is publicly available, agencies need to implement advanced security measures and protocols to ensure that this critical data remains secure.
Pushing processing power
Historically, tactical edge devices collect information and send data back to command data centers for analysis. Their limited computing and processing capabilities slow battlefield decision-making, but they don’t have to. Processing at the edge saves time and avoids significant costs by allowing devices to upload analysis results to the cloud instead of vast amounts of raw data.
However, AI at the edge requires equipment with sufficient computing power for today and tomorrow’s algorithms. Devices and sensors must be able to operate in a standalone manner to perform computing, analysis, learning, training, and inference in the field, wherever that may be. Whether on the battlefield or attached to a patient in a hospital, AI at the edge learns from scenarios to better predict and respond for the next time. For the Navy crew, that could mean identifying what path a ship of interest may take based on previous encounters. In a hospital, sensors could flag the symptoms of a heart attack before arrest happens.
Connectivity will be necessary, but systems should also be able to operate in degraded or intermittent communication environments. Using 5G or other channels allows sensors to talk and collaborate while disconnected from headquarters or a command cloud.
Another consideration is orchestration: Any resilient system should include dynamic role assignments. For example, if multiple drones are flying and the leader gets taken out, another system component needs to assume that role.
Shrinking AI to manageable size
A battlefield is not an ideal environment for artificial intelligence. AI models like ChatGPT operate in climate-controlled data centers on thousands of GPU servers that consume enormous energy. They train on massive datasets, and their computing requirements increase exponentially in operational inference stages. The scenario presents a new size, weight, and power puzzle for what the military can deploy at the edge.
Some AI algorithms are now being designed for SWAP-constrained environments and novel hardware architectures. One option is miniaturizing AI models. Researchers are experimenting with multiple ways to make smaller, more efficient models through compression, model pruning, and other options.
Miniaturization has risks. A trained model could undergo “catastrophic forgetting” when it no longer recalls something previously learned. Or it could increasingly generate unreliable information — called hallucinations — due to flaws introduced by compression techniques or training a smaller model pulled from a larger one.
Computers without borders
While large data centers can be physically walled off with gates, barriers, and guards, AI at the edge presents new digital and physical security challenges. Putting valuable, mission-critical data and advanced analytics capabilities at the edge requires more than protecting an AI’s backend API.
Adversaries could feed bad or manufactured data in a poisoning attack to taint a model and its outputs. Prompt injections could lead a model to ignore its original instructions, divulge sensitive data, or execute malicious code. However, defense-in-depth tactics and hardware features like physical access controls, tamper-evident enclosures, along with secure boot and trust execution environments / confidential computing can help prevent unauthorized access to sensitive equipment, applications, and data.
Still, having AI capabilities at the tactical edge can provide a critical advantage during evolving combat scenarios. By enabling advanced analytics at the edge, data can be quickly transformed into actionable intelligence, augmenting human decision-making with real-time information and providing a strategic advantage over adversaries.
Steve Orrin is Federal Chief Technology Officer at Intel.